Solutions & Technologies
AI Data Center Networking
Recent advances in generative artificial intelligence (AI) have captured the imaginations of hundreds of millions of people around the world and catapulted AI and machine learning (ML) into the corporate spotlight. Data centers are the engines behind AI, and data center networks play a critical role in interconnecting and maximizing the utilization of costly GPU servers that perform the compute-intensive processing in an AI training data center.
Data center networks optimized for AI/ML workloads require special capabilities around congestion management, load balancing, and more to optimize AI modeling performance and economics. The Juniper solution meets these challenges with high-capacity, scalable, non-blocking networking fabrics that deliver the highest AI performance.
Improving the economics of AI
The economics of AI training relies on advanced networking that is fast, simple, and intelligent.
How Juniper can help
Juniper innovation consistently drives new levels of scale, performance, and user experience. Our AI networking solution helps customers build high-capacity, scalable, easy-to-operate network fabrics that deliver the fastest job completion time (JCT) while maximizing GPU utilization and improving economics.
High-performance AI fabrics
Maximizing GPU utilization and minimizing idle time are key economic factors in training AI models. The Juniper AI networking solution optimizes JCT and minimizes tail latency utilizing a mix of fixed form factor, and high radix switches, combined with our broad silicon portfolio. This solution provides use case flexibility, optimized for factors such as power efficiency and scale.
Open, standards-based solution
Proprietary solutions that lock in enterprises can stifle AI innovation. Juniper firmly supports the Ethernet standard in data center networks with a robust vendor ecosystem that spurs innovation and drives down costs. Moreover, we’re committed to multivendor data center operations with our Juniper Apstra intent-based fabric management and automation software.
Experience-first operations
Data center networks are increasingly complex, requiring new protocols to meet AI workload performance demands. Automation with our Junos operating system and Juniper Apstra data center management software shields network operators from that complexity and streamlines data center operations with a multivendor, experience-first approach.
Related Solutions
Data Center Networks
Simplify operations and assure reliability with the modern, automated data center. Juniper helps you automate and continuously validate the entire network lifecycle to ease design, deployment, and operations.
Data Center Interconnect
Juniper’s DCI solutions enable seamless interconnectivity that breaks through traditional scalability limitations, vendor lock-in, and interoperability challenges.
Converged Optical Routing Architecture (CORA)
CORA is an extensible, sustainable, automated solution for IP-optical convergence. It delivers the essential building blocks operators need to deploy IP-over-DWDM transformative strategies for 400G networking and beyond in metro, edge, and core networks.
IP Storage Networking
Simplify your data storage and boost data center performance with all-IP storage networks. Use the latest technologies, such as NVMe/RoCEv2 with 100G/400G switching or NVMe/TCP, to build high-performance storage or converge your storage and data into a single network.
CUSTOMER SUCCESS
SambaNova makes high performance and compute-bound machine learning easy and scalable
AI promises to transform healthcare, financial services, manufacturing, retail, and other industries, but many organizations seeking to improve the speed and effectiveness of human efforts have yet to reach the full potential of AI.
To overcome the complexity of building complex and compute-bound machine learning (ML), SambaNova engineered DataScale. Designed using SambaNova Systems’ Reconfigurable Dataflow Architecture (RDA) and built using open standards and user interfaces, DataScale is an integrated software and hardware systems platform optimized from algorithms to silicon. Juniper switching moves massive volumes of data for SambaNova’s Datascale systems and services.
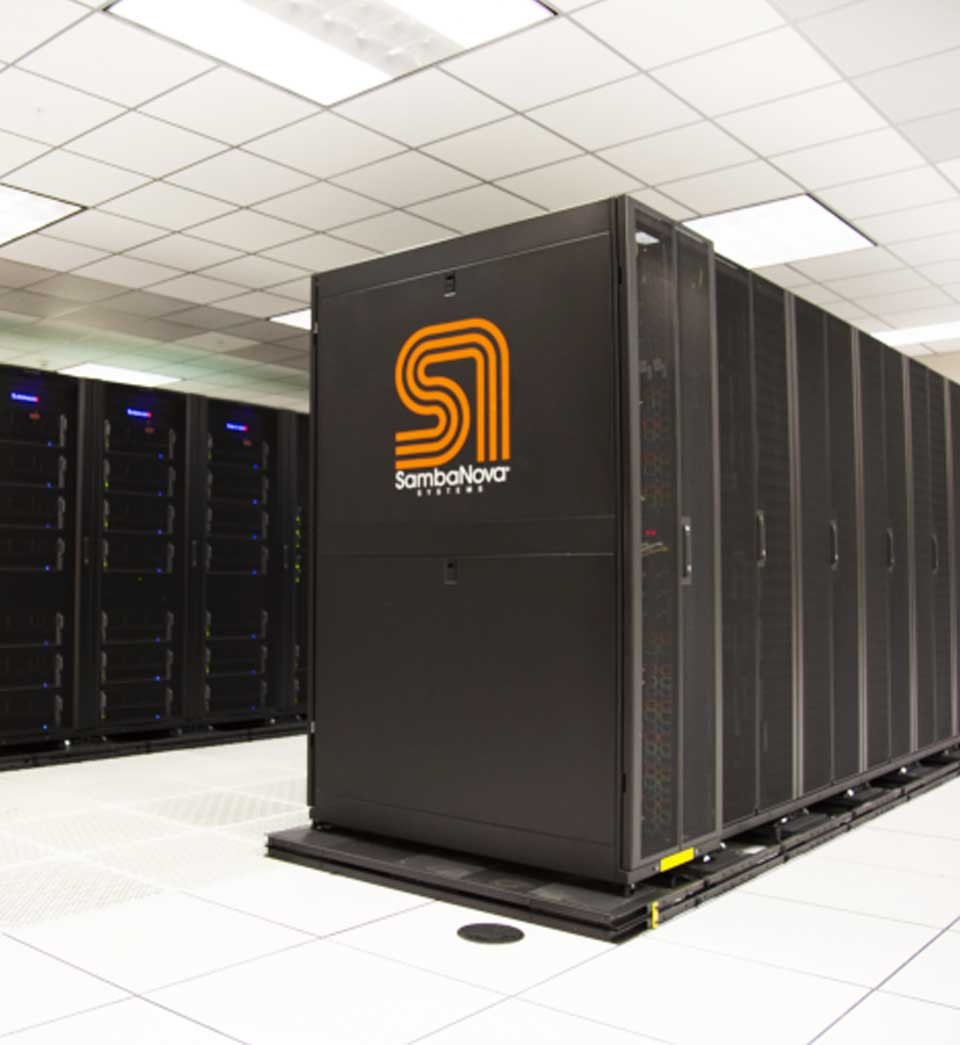
Resource Center
Whitepapers
Videos
AI Data Center Networking FAQs
What types of businesses are prioritizing the deployment of AI/ML solutions in their data centers today?
AI demand is driving hyperscalers, cloud providers, enterprises, governments, and educational institutions to incorporate AI into their business systems to automate operations, generate content and communications, and improve customer service.
What is the difference between the training and inference stages of AI?
AI models are built using carefully crafted data sets during the training stage. Training happens across multiple GPUs spanning tens, hundreds and even thousands of GPUs in a cluster - all connected across a network and constantly exchanging data with each other. After this training stage, the model is essentially complete. During the inference stage, users interact with the model, which can recognize images or generate pictures and text to provide answers to user questions. Training is typically an offline operation, whereas inference is generally online.
What are the components of AI data center network infrastructure solution, and how does Juniper enable them?
Massive AI data sets are creating the need for greater compute power, faster storage, and high-capacity, low-latency networking. Juniper helps meet these requirements in the following ways:
- Compute: AI/ML compute clusters place heavy requirements on the inter-node network. Lowering job completion time (JCT) is essential, and the network plays a key part in the efficient operation of the cluster. Juniper offers a range of high-performance, non-blocking switches with deep buffer capability and congestion management that, when architected optimally, eliminate any network bottleneck.
- Storage: In AI/ML clusters and high-performance computing, rarely can an entire data set or model be stored on the compute nodes, so a high-performance storage network is required. Juniper QFX Series Switches can be used for IP storage connectivity; they offer full support for Remote Direct Memory Access (RDMA) networking, including Non-Volatile Memory Express/RDMA over Converged Ethernet (NVMe/RoCE) and Network File System (NFS)/RDMA.
- Network: AI training models involve large, intense computations distributed over hundreds or thousands of CPU, GPU, and TPU processors. These computations demand high-capacity, horizontally scalable, and error-free networks. Juniper QFX switches and PTX Series Routers support these large computations within and across data centers with industry-leading switching and routing throughput and data center interconnect (DCI) capabilities.
How does the Juniper AI Data Center Networking solution address congestion management, load balancing, and latency requirements for maximizing AI performance?
Juniper high-performance, non-blocking data center switches provide deep buffering and congestion management to eliminate network bottlenecks. To balance traffic loads, we support dynamic load balancing and adaptive routing. For congestion management, Juniper fully supports Data Center Quantized Congestion Notification (DCQCN), Priority Flow Control (PFC), and Explicit Congestion Notification (ECN). Finally, to reduce latency, Juniper uses best-of-breed merchant silicon and custom ASIC architectures that maximize buffers where needed, virtual output queuing (VOQ), and cell-based fabrics within our spine architectures.
What does Juniper offer for IP storage?
Our portfolio includes open, standards-based switches that provide IP-based storage connectivity using NVMe/RoCE or NFS/RDMA (see earlier FAQ). Our IP Storage Networking solution designs can scale from a small four-node configuration to hundreds or thousands of storage nodes.